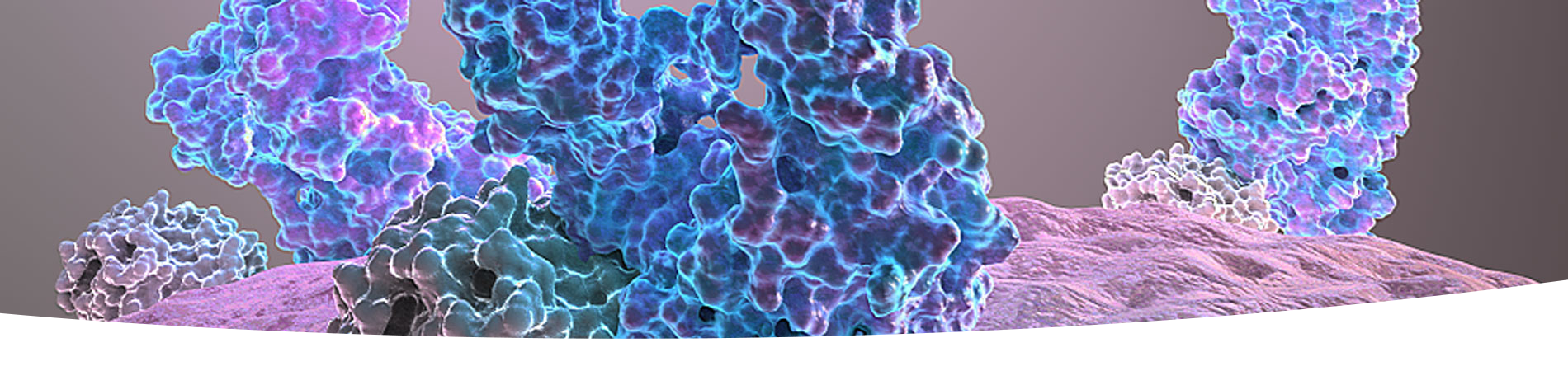
SickKids Research Institute
Driving clinical impact with every
research question
The SickKids Research Institute (RI) is Canada’s largest, hospital-based child health research institute conducting and translating groundbreaking research, training the next generation of researchers, and supporting global scientific communities with knowledge and state-of-the-art facilities.
We’re taking more discoveries from the lab bench to the bedside, making a bigger impact on children’s lives around the world, sooner.
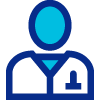
665 scientists
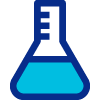
Over 1,300 research trainees
401 project & team investigators
1,253 research support staff
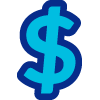
$298.6M research spend
2,794 publications
#1 research intensive hospital in Canada
#2 paediatric hospital in the world
Bridging research and care
Explore the Institute
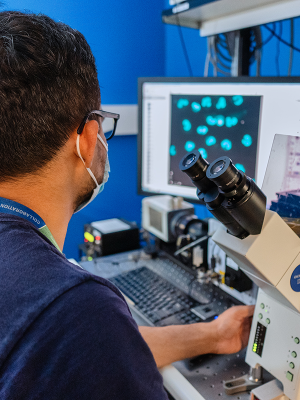
Discover world-class facilities
Enhance the scope and quality of your research. Conduct your studies in a world-class setting equipped with new technologies and infrastructure – check out our facilities and learn what our distinct research design services can offer you.
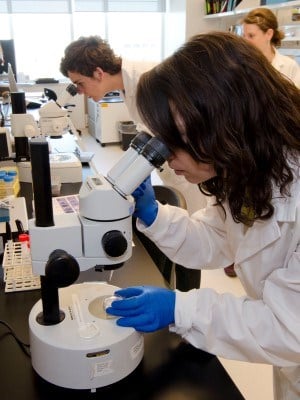
Work alongside renowned researchers
The Research Training Centre (RTC) offers trainees hands-on opportunities to contribute to some of the most important research projects in Canada and to work with top scientists.
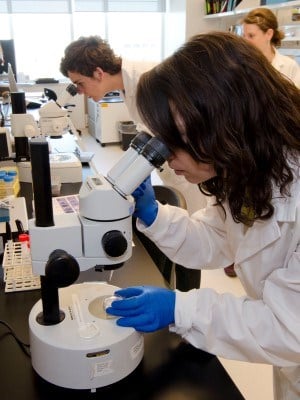
Explore leading edge research
Across our seven research programs, clinician scientists and researchers are conducting ground-breaking research to improve child health outcomes, policy and clinical care.
Latest research news
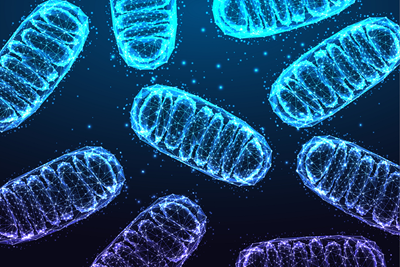
July 10, 2025
Researchers discover new way cells protect themselves from damage
International effort reveals how cell compartments form a connected defense system to maintain cellular health.

June 20, 2025
New partnership project aims to bring customizable treatments to patients with genetic conditions
This project is funded by the Precision Child Health Partnership Translational Program, part of the collaborative efforts between two of Canada’s largest child health research hospitals, SickKids and CHU Sainte-Justine. The project is powered by the catalytic philanthropy of the Azrieli Foundation and their landmark grant to both institutions in December 2023.

June 19, 2025
Transforming care through research: SickKids launches Clinical Research Strategy
SickKids has launched its first enterprise-wide Clinical Research Strategy to embed research into care, driving discovery and better outcomes for patients and families.
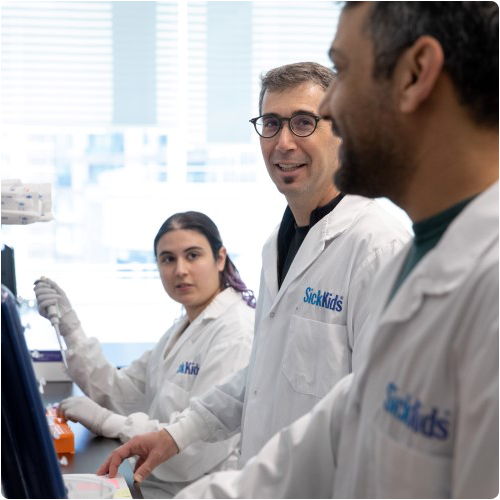
Research community
Discovery District
In the heart of Toronto’s Discovery District and affiliated with the University of Toronto, our scientists are steps away from Canada’s largest concentration of hospitals, research institutes, business incubators and venture capital organizations.
Innovation hub
Our collaborative partnerships are key to uncovering the next breakthrough in child health research. With the support of Industry Partnerships & Commercialization (IP&C), our scientists help make Toronto one of the most innovative cities in the world.
Powered by diversity
At the SickKids Research Institute, you are part of a community that has come together from across the globe to advance innovative and impactful paediatric health research.
World-renowned research
From esteemed child health researcher Dr. Zulfiqar Bhutta (winner of the 2023 Henry G. Friesen International Prize in Health Research) to the 35 scientists currently holding Canada Research Chairs, we are leaders in child health research in Canada and around the world.
More from the Research Institute
Comprised of internationally-recognized leaders in research and innovation, the SickKids Scientific Advisory & Innovation Board (SAIB) supports and provides input on the overall strategic directions for the Research Institute.
Canada Research Chairs showcase the calibre and diversity of scientists and clinicians working at the SickKids Research Institute.
SickKids is home to 59 scientific and academic chairs. See who holds current chairs in Canada.
The SickKids Institutional Research Data Management (RDM) strategy outlines our commitment to fostering sound RDM practices and supporting researchers in following the FAIR principles to make data findable, accessible, interoperable and reusable.
Research Awards & Financial Services (RAFS) supports researchers through the grant lifecycle, from grant applications and contract management, to monitoring and financial reporting.
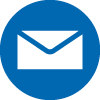
Contact the Research Institute
For all inquiries about SickKids Research Institute, please email Jelena Djurkic, Manager, Communications (Research Institute & Strategic Initiatives), Communications & Public Affairs at jelena.djurkic@sickkids.ca.